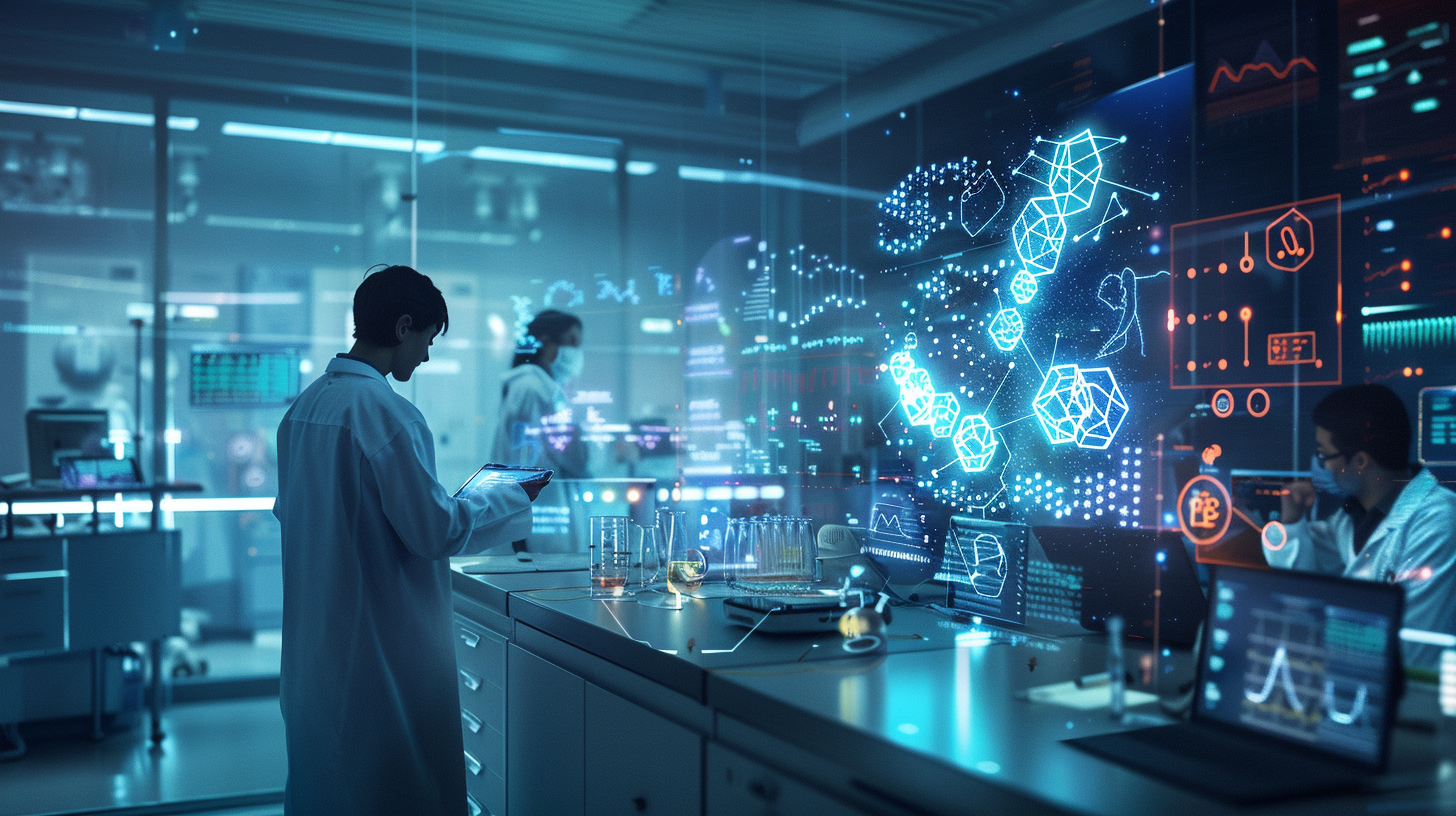
Transforming Life Science Data with Rhino Health’s Harmonization Copilot
Executive Summary
The Harmonization Copilot, a new application for the Rhino Federated Computing Platform (Rhino FCP), addresses the critical challenge of data fragmentation in the life sciences sector by automating the cleaning, standardization, and integration of diverse datasets from clinical trials, patient records, laboratory tests, and genetic sequences. Leveraging Generative AI¹, the Harmonization Copilot enhances data quality, consistency, and interoperability, enabling more efficient and accurate scientific research and development. The Harmonization Copilot offers users automated data cleaning & curation, semantic & syntactic mapping, and custom ontologies.
This innovative application supports biopharma companies in executing AI-driven projects for drug discovery, patient stratification, and personalized medicine, ultimately accelerating research timelines, reducing costs, and improving patient outcomes. By ensuring regulatory compliance and facilitating secure, collaborative data analysis, the Harmonization Copilot drives innovation and progress in the life sciences, offering a comprehensive solution for researchers and data scientists.
1. Introduction
Biopharma companies have ambitious AI agendas, aiming to leverage AI for drug discovery, patient stratification, and personalized medicine. To be effective, these initiatives require large quantities of experimental and real-world data (RWD) from multiple sources, including clinical trials, patient records, laboratory tests, and genetic sequences. These data are often stored in idiosyncratic local data models versus the sponsor’s preferred model. To use these data, sponsors must go through a previously manual, slow harmonization process, burdening data engineering teams and slowing projects. Rhino Health’s Harmonization Copilot addresses this challenge.
The Harmonization Copilot application is designed to streamline the data integration process. It automates data cleaning, standardization, and integration, enabling researchers and clinicians to work with comprehensive, reliable datasets without the usual manual effort. By transforming disparate data sources into a cohesive, standardized format, researchers can access and analyze information more efficiently, leading to faster and more accurate insights. This, in turn, accelerates drug development pipelines and safety studies.
2. The Challenge of Data Harmonization in Life Science
Data in life science comes from diverse sources, each with its unique format and structure. Clinical trials produce vast datasets encompassing patient demographics, treatment protocols, and outcomes. Patient records contain detailed medical histories, diagnostic information, and treatment outcomes. Laboratory tests add another layer of complexity with data on biochemical assays, imaging results, and genetic sequences. Genetic tests, particularly next-generation sequencing (NGS), generate terabytes of data on individual genomes, revealing genetic variations and disease mechanisms.
The sheer volume and diversity of data in life sciences present significant management challenges—data quality issues, such as missing values and inconsistencies, impact research reliability. Formatting discrepancies, where different data sources use varying terminologies, units and structures, complicate the integration, and consolidating data from incompatible systems is a significant challenge. This fragmentation hampers the ability to perform comprehensive analyses, as data must be meticulously cleaned and standardized before it can be used effectively.
The consequences of unharmonized data are far-reaching. Inefficiencies in data management lead to significant delays in research and development processes. Researchers and data scientists often spend a disproportionate amount of time cleaning and harmonizing data from different sources before analysis can begin. This reduces time spent on actual research and discovery. Errors introduced by manual data handling and inconsistencies can compromise the validity of research findings, leading to incorrect conclusions and potentially harmful clinical decisions. Furthermore, unharmonized data delays decision-making, as researchers and clinicians must sift through disjointed datasets to extract meaningful insights. This can slow down drug development timelines, impede the discovery of new treatments, and ultimately affect patient care.
Additionally, some data cannot be centralized effectively due to compliance risks (e.g., biometric data) or costs (e.g., digital pathology slides). The Harmonization Copilot advanced AI capabilities, such as Generative AI, specifically Large Language Models (LLMs), combined with Rhino FCP’s Federated Learning¹ and Edge Computing² technologies, are key to extracting value from these centralized datasets.
3. Introducing Rhino Health’s Harmonization Copilot
Harmonizing this diverse data is critical in the complex landscape of life sciences, where data comes from many sources. The Harmonization Copilot, part of the Rhino Federated Computing Platform (Rhino FCP), automates and streamlines data harmonization, transforming raw data into a coherent, actionable format, enabling more efficient and accurate scientific research and development.
At the core of the Harmonization Copilot is the integration of Generative AI, which leverages LLMs to create coherent and contextually relevant text based on vast amounts of training data, enhancing applications such as automated data harmonization and natural language processing. These technologies enable the Harmonization Copilot to understand and generate human-like text, making it capable of interpreting complex scientific terminologies and contexts. By using LLMs, the Harmonization Copilot can automatically generate accurate and standardized data descriptions, annotations, and reports, enhancing the overall quality and usability of the data.
The Rhino FCP significantly streamlines setting up a RWD network. By leveraging Federated Learning and Edge Computing technologies, the Rhino FCP allows multiple institutions to collaborate securely without centralizing sensitive data. This ensures compliance with data privacy regulations while facilitating the integration and analysis of diverse datasets. The combination of the Harmonization Copilot and Rhino FCP provides a comprehensive solution for the life science industry, enhancing data interoperability and accelerating the pace of discovery.
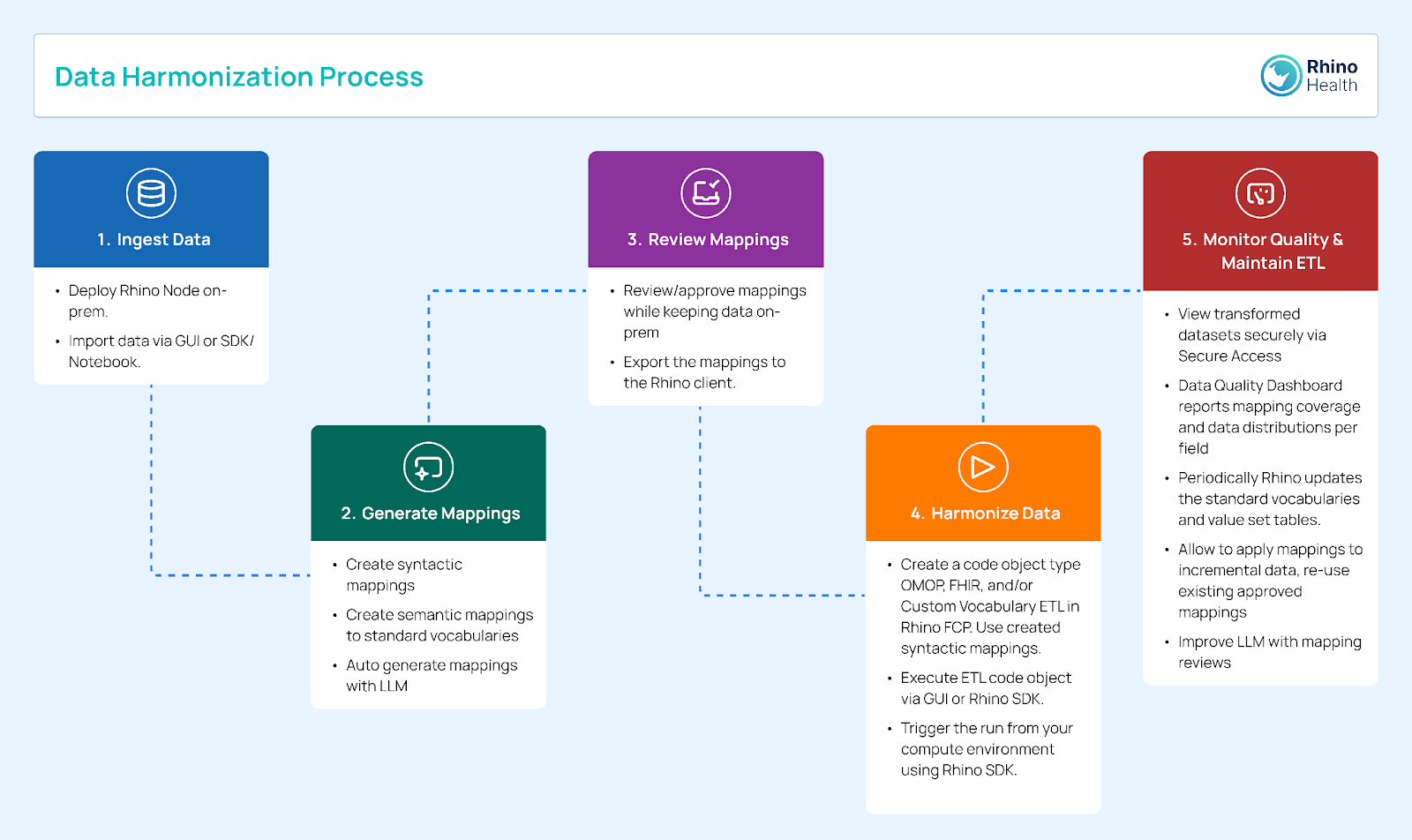
3.1. Key features and Capabilities
3.1.1. Automated Data Cleaning and Curation
The Harmonization Copilot uses Generative AI to automate data cleaning and curation. This feature significantly reduces the manual effort required to prepare data for analysis, allowing scientists to focus on high value activities such as data interpretation and hypothesis generation. By automatically identifying and rectifying inconsistencies, gaps, and errors in datasets, the Harmonization Copilot ensures that the data is accurate, complete, and ready for use in advanced analytics and machine learning applications.
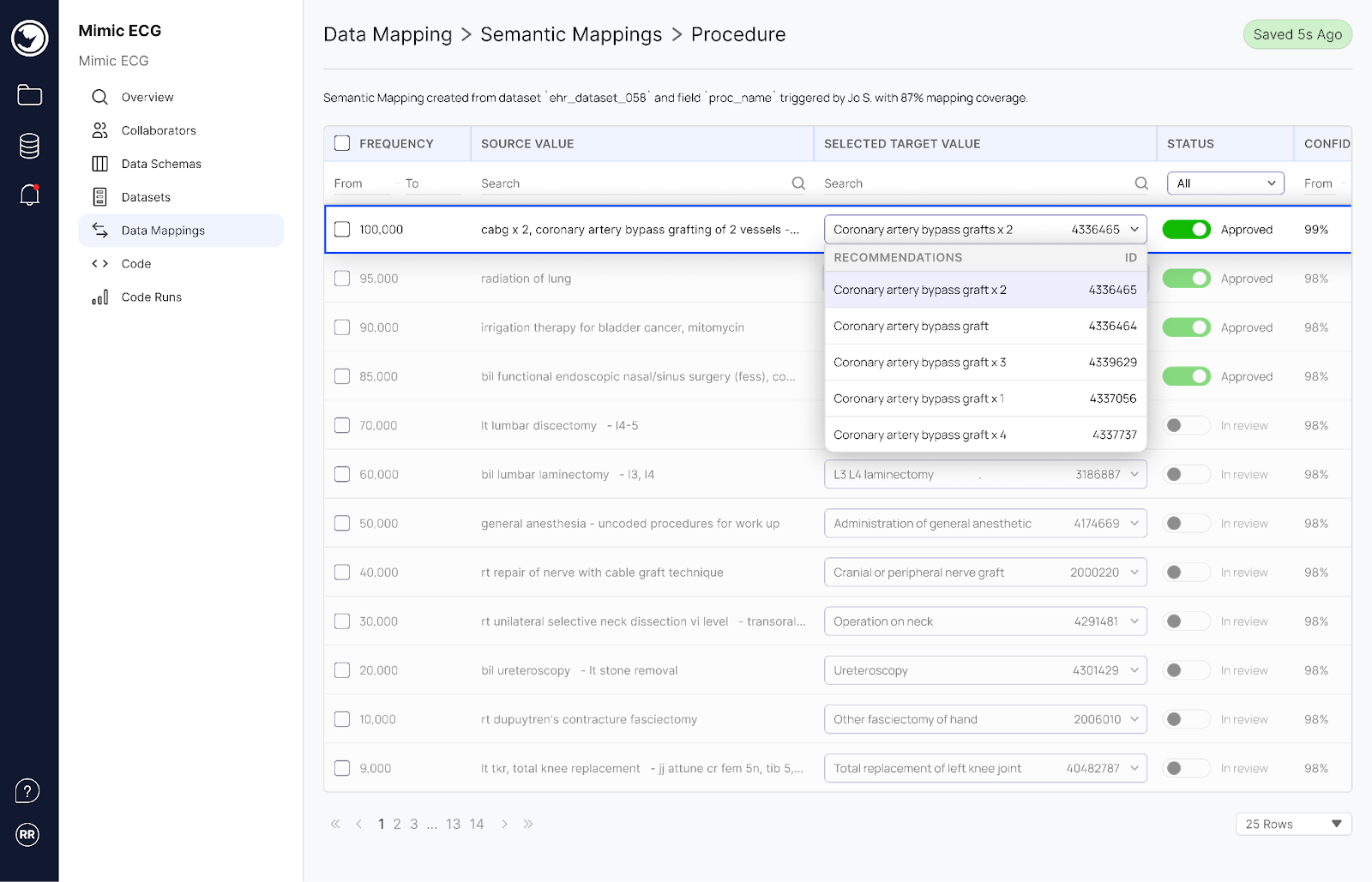
3.1.2. Semantic and Syntactic Mapping
The Harmonization Copilot can perform semantic and syntactic mapping, crucial for achieving data interoperability. Semantic mapping⁸ ensures that the meaning of the data is consistent across different sources, while syntactic mapping⁹ focuses on ensuring that data follows the correct structure and format. This dual approach allows the Harmonization Copilot to integrate diverse datasets seamlessly, providing a unified view essential for comprehensive analysis.
3.1.3. Custom Ontologies and Controlled Vocabularies
Another feature of the Harmonization Copilot is its ability to create and manage custom ontologies and controlled vocabularies. Ontologies provide a structured framework for organizing information, defining relationships between different data elements, and standardizing terminology across datasets. This capability is particularly valuable in life sciences, where varying terminologies and classifications can lead to inconsistencies and misinterpretations. Using custom ontologies, the Harmonization Copilot ensures that all data is uniformly categorized and easily interpretable, enhancing the reliability and comparability of research findings.
3.2. Integration with Rhino Federated Computing Platform (Rhino FCP)
The Harmonization Copilot is seamlessly integrated with the Rhino Federated Computing Platform . Rhino FCP enables decentralized data processing, allowing multiple institutions to collaborate on data-driven projects without sharing sensitive data directly. This integration enhances the Rhino FCP’s capabilities by providing a comprehensive solution for data harmonization.
Researchers can use the Harmonization Copilot to prepare their data within the secure, scalable, and collaborative environment of Rhino FCP, ensuring their datasets are harmonized and ready for advanced computational analysis. Integrating Generative AI and LLMs within the Harmonization Copilot significantly enhances its capabilities. The pre-filtering service narrows potential vocabulary terms using text matching before passing them to the LLM, ensuring efficient and accurate mappings. This synergy enhances the efficiency and accuracy of data processing and supports the overarching goals of accelerating scientific discovery and improving patient outcomes.
Once the data harmonization process is complete, biopharma data scientists can efficiently execute AI applications in biopharma for drug discovery, patient stratification, and personalized medicine. Integrating Generative AI and LLMs within the Harmonization Copilot further enhances these capabilities, enabling more sophisticated data analysis and insight generation.

4. Benefits of the Harmonization Copilot
Improved Data Quality and Consistency: The Harmonization Copilot significantly enhances data quality and consistency by automating the cleaning and curation process. This automation eliminates errors and inconsistencies that often arise from manual data handling. By standardizing data formats and terminologies, the Harmonization Copilot ensures that datasets are reliable and uniform, crucial for accurate analysis and reproducible results.
Enhanced Data Integration and Interoperability: One of Harmonization Copilot’s core strengths is its semantic⁴ and syntactic⁵ mapping capability. This dual approach facilitates the seamless integration of diverse datasets, making combining and analyzing information from various origins easier. As a result, researchers gain a comprehensive and unified view of their data, which is essential for in-depth analysis and discovery.
Time and Cost Savings: The Harmonization Copilot saves considerable time and resources by automating the harmonization process. Researchers no longer need to spend countless hours manually cleaning, curating, and organizing data. This time savings translates into cost savings, reducing the need for extensive manual labor and allowing research teams to allocate their resources more efficiently. Consequently, organizations can focus their efforts on higher-value activities such as data analysis and hypothesis generation.
Accelerated Research and Drug Development: With improved data quality and seamless integration, the Harmonization Copilot accelerates the pace of research and drug development. High-quality, harmonized data enables researchers to conduct more accurate and efficient analyses, leading to faster identification of potential drug candidates and biomarkers. The streamlined data management process also reduces the time required to move from data collection to actionable insights, speeding up the overall research and development timeline.
Better Patient Outcomes: Ultimately, the Harmonization Copilot contributes to better patient outcomes by enhancing the reliability and efficiency of research. High-quality, harmonized data ensures that clinical trials and research studies are based on accurate and consistent information, leading to more reliable results. This reliability is critical for developing effective treatments and interventions. Additionally, the accelerated pace of research means that new therapies and treatments can reach patients faster, improving their chances of better health outcomes.
“Overseeing our partnership with Rhino Health has been transformative. The Harmonization Copilot has changed how we handle clinical data, seamlessly integrating and standardizing vast arrays of information across multiple systems. The Rhino Federated Computing Platform’s Harmonization Copilot not only enhances our operational efficiency but also boosts our capabilities in patient care and clinical research, strengthening our healthcare innovation assets at ARC Innovation at Sheba Medical Center.” —Benny Ben Lulu, Chief Digital Transformation Officer, Sheba Medical Center and Chief Technology Officer at ARC Innovation.
5. Real-World Applications and Use Cases
5.1. Harmonizing CRO Data for Cost-Effective Collaboration
Contract Research Organizations (CROs) are crucial in conducting studies for drug development, drug efficacy, and drug safety. However, the data produced by different CROs often come in varied formats, making integration challenging and time-consuming. The Harmonization Copilot can streamline data integration for CRO collaborations. It enables companies to eliminate manual data processing steps, reduce errors, and accelerate drug development timelines by harmonizing ADME data from multiple CROs. This integration enhances efficiency and significantly cuts down costs associated with data management.
By automating data cleaning and curation, the Harmonization Copilot drastically reduces the time and resources required for data integration. Researchers can focus on high-value activities such as data analysis and hypothesis generation. The resulting harmonized data improves data quality and consistency, facilitating more accurate analyses and better decision-making in drug development.
5.2. Standardizing Clinical, Imaging, Omics, and Provider Data
Integrating clinical labs/EHRs/wearables, imaging, multi-omics, and provider master data from multiple sources poses significant challenges for research, clinical development, and real world insights. The pain points include heterogeneity in data formats, vocabularies, and inconsistent data quality. Suppose you discover a biomarker based on data from a repository and want to validate the discovered biomarkers using independent datasets from other sets of repositories. In that case, you may wish consistency across these repositories to gain confidence in the model validation. Another scenario could be longitudinal patient tracking for treatment response prediction or patient timeline insights across multiple data sources.
The Harmonization Copilot can standardize such data, transforming it into a consistent format that researchers and analysts can easily access and analyze. Its ability to create and manage custom ontologies and controlled vocabularies ensures that all data is uniformly categorized, enhancing the reliability and comparability of research findings.
The Harmonization Copilot significantly enhances data integration and interoperability by automating multimodal data standardization. This allows researchers to view their data comprehensively, which is essential for in-depth analysis and discovery. The time and cost savings from the increased efficiency and reliability of data processing accelerate research timelines and improve the quality of scientific insights.
5.3. Harmonizing Molecular and Experimental Data
Molecular and experimental data repositories can greatly enhance the efficiency and precision of research and development by performing feature selection, statistical modeling, and AI modeling. These models require high-quality data accompanied by standard metadata. However, the data repositories often have messy data formats, usually not annotated, and lack standard data formats and terminologies, making it challenging to harmonize and integrate these diverse datasets. This hampers the ability to draw meaningful insights, leading to delayed decision-making. The Harmonization Copilot can transform fragmented data into a cohesive, actionable format. This harmonization improves data quality and consistency, enabling more accurate analyses and accelerating drug development processes, facilitating timely decision-making.
The Harmonization Copilot enhances the scalability and reliability of data management. Ensuring that all data is consistent and accurate supports more reliable research findings and better decision-making. The automated harmonization process saves considerable time and resources, allowing the company to focus on innovative research and development efforts.
To see the Harmonization Copilot in action and learn more about its real-world impact, read our Case Study: Automating Clinical Data Standardization for ARC Innovation at Sheba Medical Center with Rhino Health’s Harmonization Copilot.
6. Conclusion
The Harmonization Copilot, part of the Rhino Federated Computing Platform, represents a significant advancement in data management for the life sciences sector. It enables researchers and clinicians to work with harmonized, reliable datasets. This collaborative environment, enhanced by Generative AI and Large Language Models (LLMs), accelerates scientific discovery and innovation.
With key features like automated data cleaning and semantic & syntactic mapping, the Harmonization Copilot is a game-changer in data harmonization. It streamlines the process, improving data quality and allowing biopharma companies to leverage AI more efficiently for drug discovery, patient stratification, and personalized medicine.
The importance of the Harmonization Copilot is evident. In an era of exponential data growth, harmonizing and integrating diverse datasets is crucial for advancing research and improving patient outcomes. It drives innovation and progress in life sciences by reducing the burden on data engineering teams and facilitating more accurate and efficient analyses.
Combined with Rhino FCP’s robust infrastructure, the Harmonization Copilot provides an unparalleled tool for researchers and data scientists. It accelerates research timelines, ensures regulatory compliance, and improves healthcare outcomes.
We invite researchers, data scientists, engineers, clinical data management professionals, and other life science stakeholders to explore the Harmonization Copilot. View our detailed documentation, the video demo, and the case study, all linked above. Contact Rhino Health today to discover how the Harmonization Copilot can elevate your data harmonization efforts and drive innovation in your organization.
References and Notes:
¹ Federated Learning: A machine learning approach where models are trained collaboratively across multiple decentralized devices or servers holding local data samples without exchanging their data. This technique enhances data privacy and security by keeping data localized and only sharing model updates.
² Edge Computing: Refers to processing data near the source generation (i.e., at the network’s edge) rather than relying on a centralized data-processing warehouse. This reduces latency, conserves bandwidth, and enhances the speed and responsiveness of data-intensive applications.
³ ETL (Extract, Transform, Load): A process that involves extracting data from various sources, transforming it into standardized formats compatible with common data models like OMOP and FHIR, and loading the harmonized data into a centralized repository. This ensures seamless data integration and enables effective data analysis.
⁴ OMOP (Observational Medical Outcomes Partnership): OMOP is a common data model designed to standardize the structure and content of observational data to facilitate analysis and improve the reliability of research findings. It is widely used in healthcare and life sciences to integrate and analyze data from disparate sources. The Harmonization Copilot supports OMOP to ensure that data from different sources can be standardized into a common format, making integrating and analyzing large datasets from diverse healthcare settings easier.
⁵ FHIR (Fast Healthcare Interoperability Resources): FHIR is a standard for exchanging healthcare information electronically. Developed by HL7, it aims to simplify the implementation of healthcare data exchange by providing a set of resources and an application programming interface (API) that supports interoperability between different healthcare systems. The Harmonization Copilot leverages FHIR to facilitate the seamless exchange of healthcare data across various systems, enhancing interoperability and enabling efficient data sharing and integration in real-time.
⁶ HIPAA (Health Insurance Portability and Accountability Act): HIPAA is a U.S. law enacted in 1996 that establishes national standards to protect sensitive patient health information from being disclosed without the patient’s consent or knowledge. HIPAA allows covered entities to share data with business associates for data aggregation and analysis, provided the data is de-identified to mitigate privacy risks.
⁷ GDPR (General Data Protection Regulation): GDPR is a comprehensive data protection law implemented by the European Union in 2018. It governs the collection, use, and transfer of personal data, including health data, within the EU and EEA. GDPR mandates strict consent requirements and data protection measures, ensuring that personal data is processed lawfully, transparently, and securely. For data harmonization in the Life Science sector, GDPR emphasizes the need for explicit consent and robust anonymization techniques to protect individual privacy.
⁸ Semantic Mapping: The process of aligning the meanings of data elements from different sources to a common vocabulary or standard. This ensures that data values are interpreted consistently, regardless of source, by translating local terminologies into standardized codes like those in SNOMED (Systematized Nomenclature of Medicine) or OMOP (Observational Medical Outcomes Partnership).
⁹ Syntactic Mapping: The process of aligning data structures and formats from different sources to a common data model. This involves ensuring that data fields match in terms of format and structure, making it possible to integrate data seamlessly across various systems.