
Transforming Data Engineering and Integration Across Sectors with Rhino Health’s Harmonization Copilot
1. Introduction
Integrating and analyzing diverse datasets is crucial for advancing research and improving health outcomes. However, the sheer volume and variety of these data generated present significant challenges. This article explores the concept of data harmonization, its importance, and how Rhino Health’s Harmonization Copilot, integrated with the Rhino Federated Computing Platform (Rhino FCP), addresses these challenges. By automating the cleaning, standardization, and integration of varied datasets, the Harmonization Copilot enhances data quality, consistency, and interoperability, ultimately driving innovation and improving patient outcomes.
2. Understanding Data Harmonization
Data harmonization ensures that data from different sources are unified into a consistent format, enabling effective cross-institution patient care, medical research, and other collaborative efforts. Harmonization is a prerequisite for effectively generating large, high-quality datasets from multiple sources needed to train AI models. Without harmonization, data collected from various organizations—and sometimes from different countries—remains fragmented, leading to inconsistencies and potential errors in analysis and research findings. Data harmonization is a critical precursor to achieving interoperability across the participants in the healthcare ecosystem.
To fully grasp the concept of data harmonization, it’s helpful to distinguish it from related processes such as data standardization and data normalization:
- Data Harmonization: Involves transforming and integrating diverse data sources into a cohesive, comparable format. Harmonization ensures that all data elements are compatible, which is essential for large-scale data analysis and AI training. For example, converting weight measurements from pounds to kilograms across datasets from different countries.
- Data Standardization: Refers to transforming data into a common format using consistent units and structures. This process ensures that data adheres to predefined standards, making it easier to compare and combine. For instance, using a single data format (e.g., YYYY-MM-DD) across all records is data standardization.
- Data Normalization: Involves organizing data to minimize redundancy and improve data integrity. It typically applies to database management, ensuring data is stored efficiently and consistently. An example of normalization is structuring a database to store customer information in one table and order details in another, linked by a unique customer ID.
The distinctions and importance of these processes are well-documented in scientific literature. For instance, Papez et al. (2023)¹ highlight the critical role of data harmonization in ensuring interoperability and facilitating large-scale analysis in their work on transforming the UK Biobank data to the OMOP² Common Data Model. Similarly, Wilkinson et al. (2016)³ emphasize the importance of standardizing data to adhere to the FAIR⁴ principles—Findable, Accessible, Interoperable, and Reusable. Data normalization principles, discussed in-depth by Codd (1970)⁵ in his pioneering work on the relational database model, remain fundamental to maintaining data integrity and reducing redundancy.
These processes—harmonization, standardization, and normalization—are interdependent and collectively ensure that data is high-quality, reliable, and ready for advanced analytical applications such as AI and machine learning. Harmonization is the most encompassing of the three, addressing the broader challenge of making diverse data sources compatible and comparable.
In the next section, we will explore the specific challenges of data harmonization and how the Harmonization Copilot effectively addresses these issues.
3. The Challenges of Data Fragmentation
Data fragmentation is a pressing issue in healthcare, life sciences, and public health. The vast amount of data generated from various sources, such as clinical trials, patient records, laboratory tests, and genetic sequences, present both an opportunity and a challenge. Understanding these challenges is crucial for leveraging data effectively.
3.1. Diversity of Data
In the healthcare, life sciences, and public health sectors, data comes from various sources and formats, necessitating harmonization:
- Patient Records: Electronic Health Records (EHRs) hold detailed medical histories, diagnostic information, and treatment outcomes. Different healthcare providers use various systems, leading to inconsistent data formats.
- Clinical Trials: Extensive datasets from clinical trials include patient demographics, treatment protocols, and outcomes, with significant variations in data formats between studies and institutions.
- Laboratory Tests: Lab results encompass biochemical assays, imaging results, and genetic sequences, often stored in proprietary formats unique to specific laboratory equipment and software.
- Genetic Sequences: Next-generation sequencing (NGS) generates vast amounts of genomic data, revealing genetic variations and disease mechanisms. This data is complex and requires specialized formats for analysis.
3.2. Impact of Unharmonized Data on Research Efficiency and Accuracy
Data stored in local formats introduces friction in data collaborations, leading to inefficiencies and inaccuracies, compliance risks, and cost issues in research:
- Time-Consuming Data Cleaning: Researchers spend a significant portion of their time cleaning and manually harmonizing data. This manual effort reduces the time available for actual analysis and discovery.
- Inconsistent Data Quality: Disparate data formats and terminologies introduce errors and inconsistencies, compromising the validity of research findings and potentially leading to incorrect conclusions.
- Delayed Decision-Making: Fragmented data delays decision-making as researchers must sift through disjointed datasets to extract meaningful insights. This slows down drug development timelines and impedes the discovery of new treatments.
- Limited Data Usability: Valuable data needs to be utilized with proper harmonization. The inability to integrate diverse datasets limits the scope of research and comprehensive analysis.
- Compliance Risks: Data privacy regulations like HIPAA⁶ and GDPR⁷ require strict adherence to data handling and storage protocols. Unharmonized data increases non-compliance risk, potentially leading to legal and financial penalties.
- Cost Issues: Cleaning and harmonizing data manually is resource-intensive and requires significant time, labor, and technology investment. For biopharma companies and healthcare organizations, the cost of managing fragmented data can be substantial, diverting resources aways from core research activities and innovation.
The following section will explore how Rhino Health’s Harmonization Copilot addresses these challenges and provides a comprehensive data integration and harmonization solution.
4. Introducing the Harmonization Copilot
Data harmonization is crucial in ensuring that diverse datasets can be effectively used for advanced analytics and research. The Harmonization Copilot, an innovative application integrated with the Rhino FCP, addresses this need by automating the cleaning, standardization, and integration of varied datasets. This section provides an overview of the Harmonization Copilot, highlighting its key features and capabilities.
The Harmonization Copilot is designed to streamline data harmonization processes. It uses Generative AI⁸ to automate the labor-intensive tasks such as cleaning, standardizing, and integrating datasets from various sources, such as clinical trials, patient records, laboratory tests, and genetic sequences. Integration with Rhino FCP ensures a secure, scalable, and collaborative environment for data harmonization that does not require centralizing data to process it.
4.1. Key Features and Capabilities
The Harmonization Copilot offers several key features and capabilities:
- Automated Data Cleaning and Curation: Harnessing advanced AI algorithms, the Harmonization Copilot automates the tedious tasks of data cleaning and curation. This capability reduces manual effort and empowers researchers to concentrate on high-value activities like data interpretation and hypothesis generation. The result is accurate, complete, and ready-for-analysis data for advanced analytics and machine learning applications.
- Semantic and Syntactic Mapping: Ensures data consistency across different sources by focusing on data’s meaning (semantic) and structure (syntactic) of data. This integration provides a unified view essential for comprehensive analysis.
- Custom Ontologies and Controlled Vocabularies: Allows for creating and managing custom ontologies and controlled vocabularies. This structured framework organizes information, defines relationships between data elements, and standardizes terminology, ensuring uniform data categorization and enhancing research reliability.
- Custom Data Hierarchies: The Harmonization Copilot streamlines data organization and retrieval by structuring data classification based on specific research needs. This feature enables researchers to access and utilize relevant information quickly and significantly accelerates the research process, saving valuable time.
- User-Friendly GUI: The human-in-the-loop workflow facilitated by GUI adds significant value, ensuring accuracy through expert oversight and adjustments. This feature supports users in visualizing and interacting with the data harmonization process seamlessly.
“Overseeing our partnership with Rhino Health has been transformative. The Harmonization Copilot has changed how we handle clinical data, seamlessly integrating and standardizing vast arrays of information across multiple systems. The Rhino Federated Computing Platform’s Harmonization Copilot not only enhances our operational efficiency but also boosts our capabilities in patient care and clinical research, strengthening our healthcare innovation assets at ARC Innovation at Sheba Medical Center.” —Benny Ben Lulu, Chief Digital Transformation Officer, Sheba Medical Center and Chief Technology Officer at ARC Innovation.
5. Workflow of the Harmonization Copilot
The Harmonization Copilot is designed to streamline and automate the data harmonization process, ensuring diverse datasets can be effectively integrated for advanced research and analysis.
Here is a step-by-step guide to how the Harmonization Copilot achieves this:
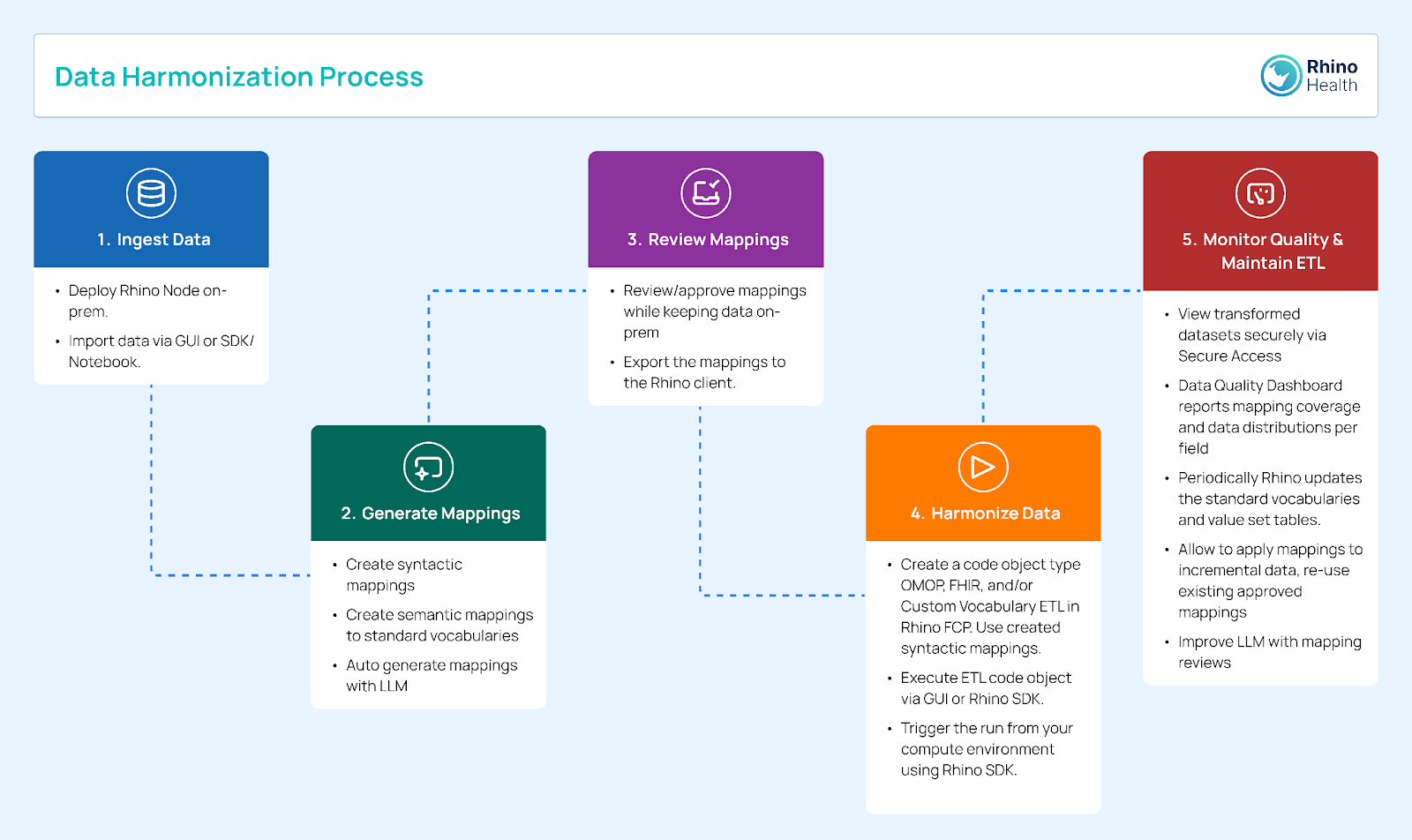
- Ingest data: First, deploy the Rhino Node on-premises to ensure data remains secure within the organization’s infrastructure. This node acts as a local gateway for data processing, minimizing data transfer and enhancing privacy and security. Then, data can be imported through a user-friendly graphical user interface (GUI) or via Software Development Kit (SDK), allowing researchers to choose the most convenient method for data ingestion, whether through the GUI or programmatically via the SDK.
- Generate Mappings: After data ingestion, create syntactic mappings to ensure data follows the correct structure and format. Develop semantic mappings to standard vocabularies to maintain consistency in the meaning of data across different sources. The Harmonization Copilot uses advanced AI to auto-generate these mappings with LLMs to enhance efficiency, ensuring accurate and automated data harmonization.
- Review Mappings: Once the mappings are generated, review and approve them while keeping data on-premises to ensure privacy and security. After the review, export the approved mappings to the Rhino Client for further use, facilitating a seamless transition to the next stage of the process.
- Harmonize Data: Utilize the created syntactic mappings to set up a code object type for OMOP, FHIR⁹¹⁰, and Custom Vocabulary ETL¹⁰ in Rhino FCP, ensuring data compatibility with industry standards. Execute the ETL code object through the GUI or programmatically via the Rhino SDK, allowing for flexible execution methods. Access the transformed datasets securely via Secure Access, ensuring only authorized users can view the harmonized data. For seamless integration, trigger the entire process from your compute environment using the Rhino SDK.
- Monitor Quality & Maintain ETL: Finally, ensure the continuous quality and maintenance of the ETL process. View transformed datasets securely via Secure Access, where the Data Quality Dashboard reports mapping coverage and data distributions per field, providing insights into data quality and mapping accuracy. Periodically, the Harmonization Copilot updates the standard vocabulary and value set tables, keeping the system aligned with the latest standards. Apply mappings to incremental data and reuse existing approved mappings to facilitate ongoing data harmonization efforts. Continuously enhance the LLMs’ accuracy and efficiency based on user feedback, ensuring the system remains practical and up-to-date.
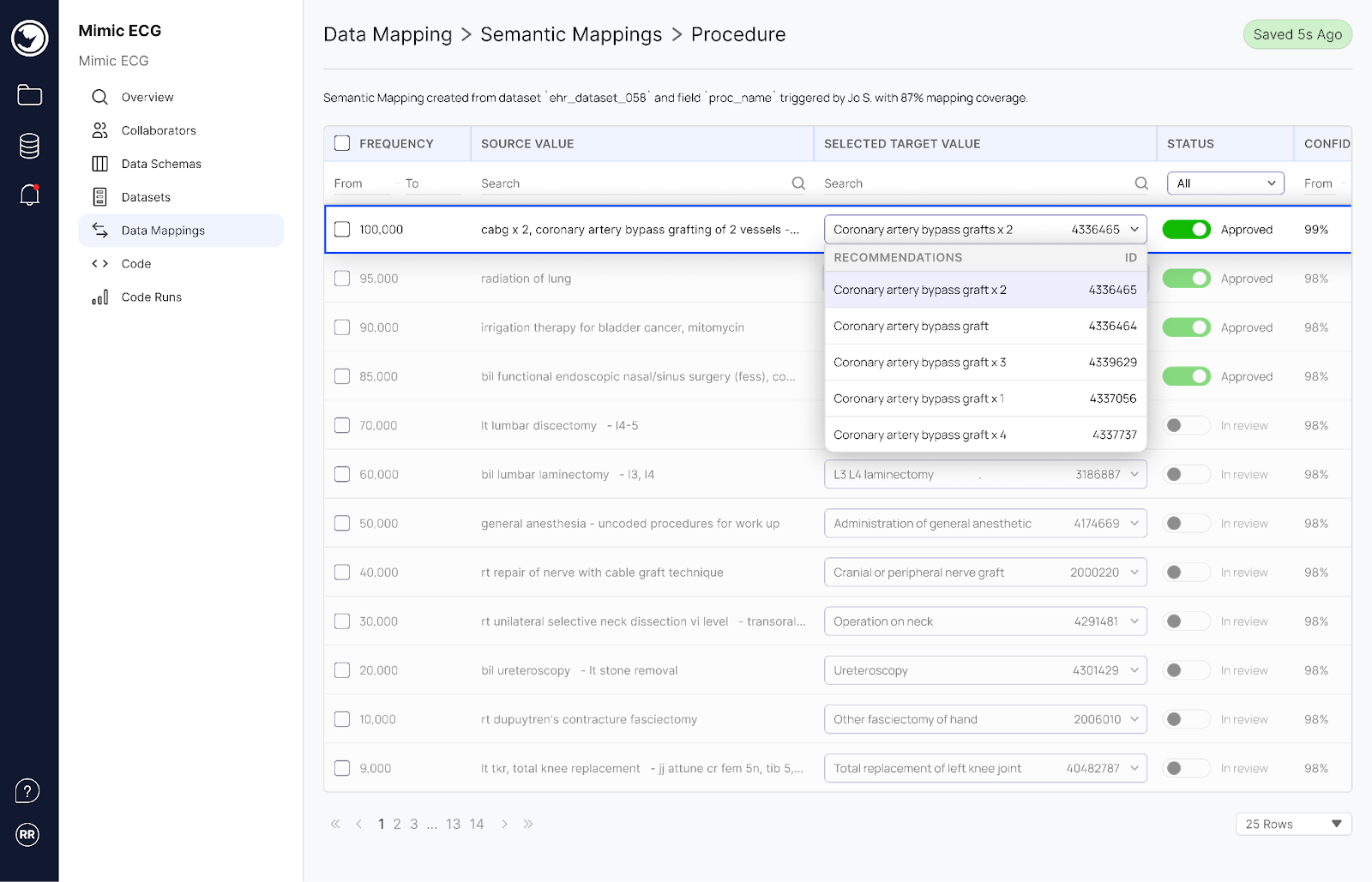
6. Integration with Rhino Federated Computing Platform (Rhino FCP)
6.1. Overview of Rhino FCP
The Rhino FCP is a robust, cloud-based solution that facilitates collaborative data analysis while ensuring data privacy and regulatory compliance. It uses Federated Learning¹¹ and Edge Computing¹³ technologies, allowing multiple institutions to collaborate on data-driven projects without directly sharing sensitive data. This approach ensures data privacy and security, and facilitates compliance with regulations like GDPR and HIPAA.
6.2. Enhancing Data Harmonization and Collaboration
Integrating the Harmonization Copilot with Rhino FCP significantly enhances data management by automating and streamlining the harmonization process at the edge. Keeping data within each organization’s secure infrastructure mitigates privacy risks and ensures compliance with regulations like HIPAA and GDPR. This decentralized method allows diverse datasets from various sources to be efficiently cleaned, standardized, and integrated without centralizing sensitive data. The Harmonization Copilot reduces manual effort and enables the creation of Federated Datasets, which can be used in collaborative research without sharing raw data.
The value chain from harmonized data to Federated Datasets to Federated Learning is transformative. Once data is harmonized at the edge, it can be used in Federated Learning, where machine learning models are trained across multiple institutions without moving the data. This ensures data privacy and enhances the robustness of the models by leveraging diverse datasets. Rhino FCP and the Harmonization Copilot support secure data access, quality monitoring, and controlled data sharing, fostering collaboration while maintaining complete control over data. This integrated approach drives innovation, improves research outcomes, and ensures transparent, compliant, and secure data processing across sectors.
6.3. Harmonization Copilot Reference Architecture within Rhino FCP

The Harmonization Copilot architecture includes the customer network with source data form databases, object storage, data lakes, FHIR interface engines and DICOM servers, alongside the Rhino Client (on-premises), which features internal storage, a data processing executor, standard vocabularies filters, and a mapping LLM.
Data engineers and clinical data SMEs access the Harmonization Copilot via the Rhino GUI. which includes managed and standard ETLs, data quality monitoring, mapping LLM fine-tuning, mapping generation and review, custom vocabularies creation, and a repository of approved mappings.
The Harmonization Copilot architecture integrates on-premises data sources with processing tools ensuring data privacy and security while facilitating efficient harmonization. It enables organizations to leverage their data for AI and machine learning applications, driving innovation and improving patient outcomes in the healthcare, life sciences, and public health sectors.
7. Benefits of Using Harmonization Copilot
The Harmonization Copilot, integrated with the Rhino FCP, addresses the data harmonization challenges in the healthcare, life sciences, and public health sectors.
- Improved Data Quality and Consistency: The Harmonization Copilot significantly enhances data quality and consistency by automating the data cleaning and curation processes. Advanced AI algorithms identify and rectify inconsistencies, gaps, and errors in datasets, ensuring the data is accurate, complete, and reliable. This automation reduces the likelihood of human error, which is critical for maintaining high standards in research and clinical studies.
- Enhanced Data Integration and Interoperability: The tool integrates diverse data sources. Using semantic and syntactic mapping, the Harmonization Copilot ensures that data from different origins can be seamlessly combined into a unified format. This integration is crucial for comprehensive analysis, allowing researchers to draw meaningful insights from a broader, more diverse dataset. This results in a more cohesive and interoperable data environment, supporting advanced analytics and AI applications.
- Time and Cost Savings: Automating the harmonization process translates into significant time and cost savings for data engineering, data science, and research teams. Traditionally, data harmonization is a labor intensive task requiring substantial manual effort. The Harmonization Copilot reduces this burden, helping data engineering, data science, and research teams to focus on higher value activities such as data interpretation and hypothesis generation. Reducing manual labor also leads to lower operational costs, making data management more efficient and cost-effective.
9. Conclusion
Data Harmonization is vital for transforming diverse datasets into a cohesive format, essential for advanced analytics and research in the healthcare, life sciences, and public health sectors. The Harmonization Copilot offers substantial benefits by automating data cleaning, standardization, and integration improving data quality, consistency, and interoperability. Unlike standardization (consistent formats) and normalization (reducing redundancy), harmonization ensures compatibility across diverse data sources.
The Rhino Federated Computing Platform’s Harmonization Copilot application significantly reduces the manual effort required for data preparation, enabling researchers to focus on high-value tasks like data interpretation and hypothesis generation. The Harmonization Copilot is invaluable for any organization dealing with complex data by enhancing research efficiency, accelerating drug development, and improving patient outcomes.
Dive into our in-depth articles, “Transforming Public Health Practice with Rhino Health’s Harmonization Copilot” and “Transforming Life Science Data with Rhino Health’s Harmonization Copilot,” to see how this technology is making an impact in the real world. To experience the capabilities of the Harmonization Copilot firsthand, schedule a demo with our team.
References and Notes:
¹ Papez, V., Denaxas, S., Hemingway, H., et al. (2023). Transforming and evaluating the UK Biobank to the OMOP Common Data Model for COVID-19 research and beyond. Oxford Academic | JAMIA A Scholarly Journal of Informatics in Health and Biomedicine. Available at: https://academic.oup.com/jamia/article/30/1/103/6760234. Accessed: 27 June 2024.
² OMOP (Observational Medical Outcomes Partnership): A standardized data model to facilitate large-scale analytics across diverse healthcare databases.
³ Wilkinson, M.D., Dumontier, M., Aalbersberg, I.J., et al. (2016). The FAIR Guiding Principles for scientific data management and stewardship. Scientific data, 3(1), pp.1-9. Available at: https://www.nature.com/articles/sdata201618. Accessed 27 June 2024.
⁴ FAIR Principles: Guidelines to improve the Findability, Accessibility, Interoperability, and Reusability of digital assets, ensuring data is discoverable, accessible, compatible, and reusable for future research.
⁵ Codd, E.F. (1970). A Relational Model of Data for Large Shared Data Banks. Communications of the ACM, 13(6), pp.377-387. Available at: https://dl.acm.org/doi/10.1145/362384.362685. Accessed 27 June 2024.
⁶ HIPAA (Health Insurance Portability and Accountability Act): A US law designed to provide privacy standards to protect patients’ medical records and other health information.
⁷ GDPR (General Data Protection Regulation): A regulation in EU law on data protection and privacy in the European Union and the European Economic area.
⁸ LLMs (Large Language Models): A type of AI model capable of understanding and generating human-like text based on large datasets.
⁹ FHIR (Fast Healthcare Interoperability Resources): A standard describing data formats and elements (known as “resources”) and an application programming interface for exchanging electronic health records.
¹⁰ ETL (Extract, Transform, Load): A database usage and data warehousing process responsible for pulling data out of the source systems and placing it into a data warehouse.
¹¹ Federated Learning: Federated Learning is a decentralized machine learning approach enabling multiple institutions to train AI models collaboratively without sharing raw data. This method ensures data privacy and security by keeping data within local environments and only sharing model updates. A prominent example of its application is the EXAM model¹² developed for predicting COVID-19 clinical outcomes, which utilized data from 20 institutes globally without direct data sharing. This model demonstrated significant improvements in predicting performance and generalizability across diverse datasets, showcasing the potential of Federated Learning in healthcare.
¹² Dayan, I., Roth, H. R., Zhong, A., et al. (2021). Federated Learning for Predicting Clinical Outcomes in Patients with COVID-19. Nature Medicine. Available at: https://www.nature.com/articles/s41591-021-01506-3. Accessed 2 July 2024. Dr. Ittai Dayan is the Co-founder and CEO of Rhino Health.
¹³ Edge Computing: A distributed computing paradigm that brings computation and data storage closer to the data sources. This approach reduces latency, enhances data security, and improves response times by processing data locally on devices or edge servers rather than relying on a centralized cloud infrastructure. Edge Computing is essential for real-time processing and is particularly beneficial in scenarios where data privacy and quick decision-making are critical.